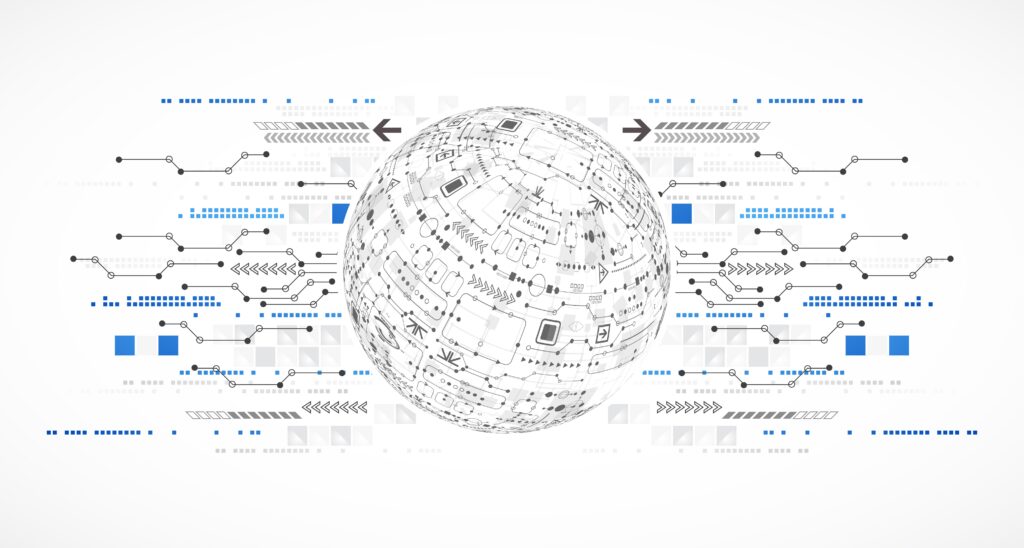
by Dirk Schmücker and Julian Reif
Paper: Measuring tourism with big data? Empirical insights from comparing
passive GPS data and passive mobile data. In: Annals of Tourism Research Empirical Insights 3(2022)
Currently, there are two valid approaches for measuring tourism frequencies and flows: (a) locally installed one-spot sensors and (b) tracking solutions based upon signal chains coming from GNNS-equipped smartphones or the mobile network they are connected to. There are some more variants of data-sources, for example, „mini-signal chains“ (constructed from local sensors catching the Bluetooth or wifi signal or public social media postings) or using water consumption in a destination, but basically, there are these two approaches: Local sensors and smartphone-based tracking. In this article, we deal with smartphone-based tracking data.
In the first step, we tried to identify and classify the different data sources relevant to tourism research. For this purpose, we propose four categories in the paper:
- Cat. A: Multi-Spot Measurements
- Cat. B: Coupled Spots
- Cat. C: Single Spot Measurements
- Cat. D: Other Measurements
In order to better compare the data sources, we have also developed a set of 13 evaluation indicators summarized in four dimensions (Figure 1):
Figure 1: Categories of data sources
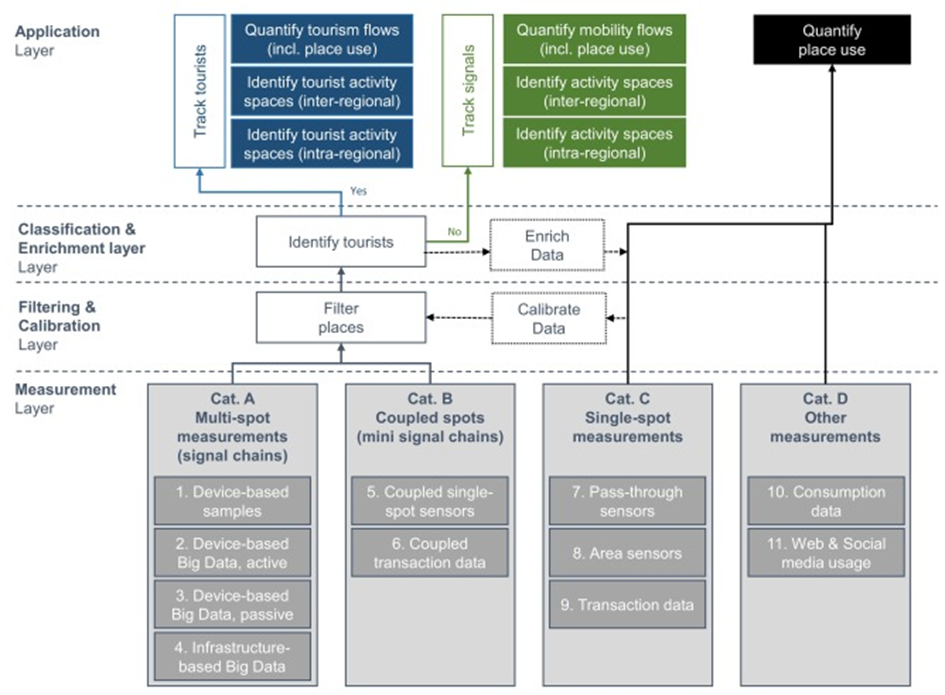
- Specific tourist dimensions
- Time and Space
- Generic dimensions
- Social and organizational dimensions
In addition to this more theoretical look at the subject, we work with empirical data and more precise Tracking Data. Tracking data are Big Data in terms of being 3V and also in terms of being „exhaust data“: These data are not being generated with the goal of tracking users, but for billing, technical operations or finding one’s place to get the correct weather forecast. However, once the data is there, why not reuse them? This idea has turned into a vibrant industry, and datasets are commercialized for considerable sums of money.
There is a growing body of academic research on such data sources, and researchers usually do fancy things within the data, mostly applying advanced ARIMA models or some machine learning algorithms. We were more interested in the relation of the data sources to the real world: Would they be able to reflect the results from reference data sources, and would they be able to identify different types of mobility?
Therefore, we use two data sources (passive mobile phone data and passive GPS location events) to get empirical insights on day and overnight tourists in four different destinations in Schleswig-Holstein, Germany (St. Peter-Ording, Büsum, Amrum, Multimar Wattforum). We compare Big Data with local reference data from tourist destinations. Figure 2 shows an example of a visual comparison of different data sources for overnight visitors. Results show that mobile network data are on a plausible level compared to the local reference data and are able to predict the temporal pattern to a very high degree. GPS app-based data also perform well but are less plausible and precise than mobile network data.
Figure 2: An example of comparing different data sources for overnight tourists
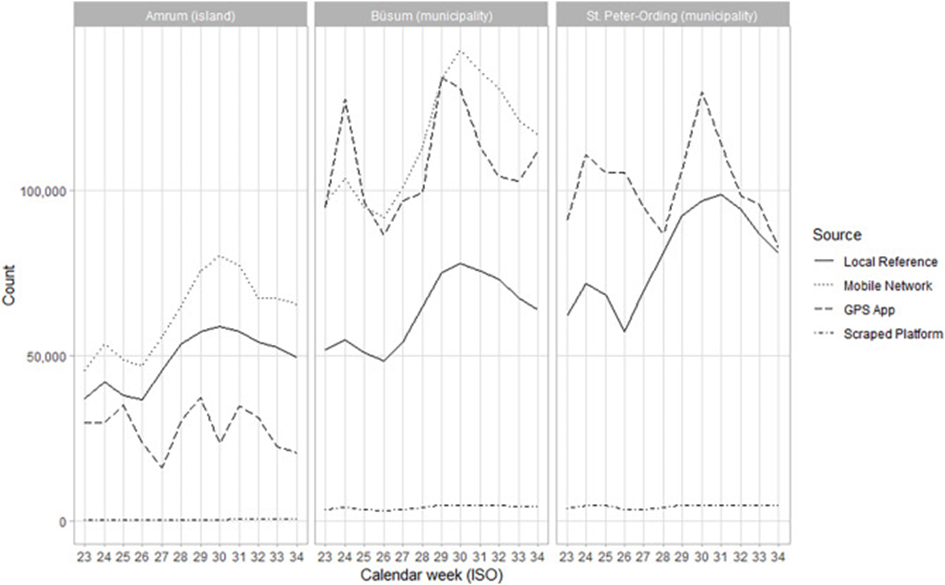
Here is a link to the open access paper: https://www.sciencedirect.com/science/article/pii/S2666957922000295