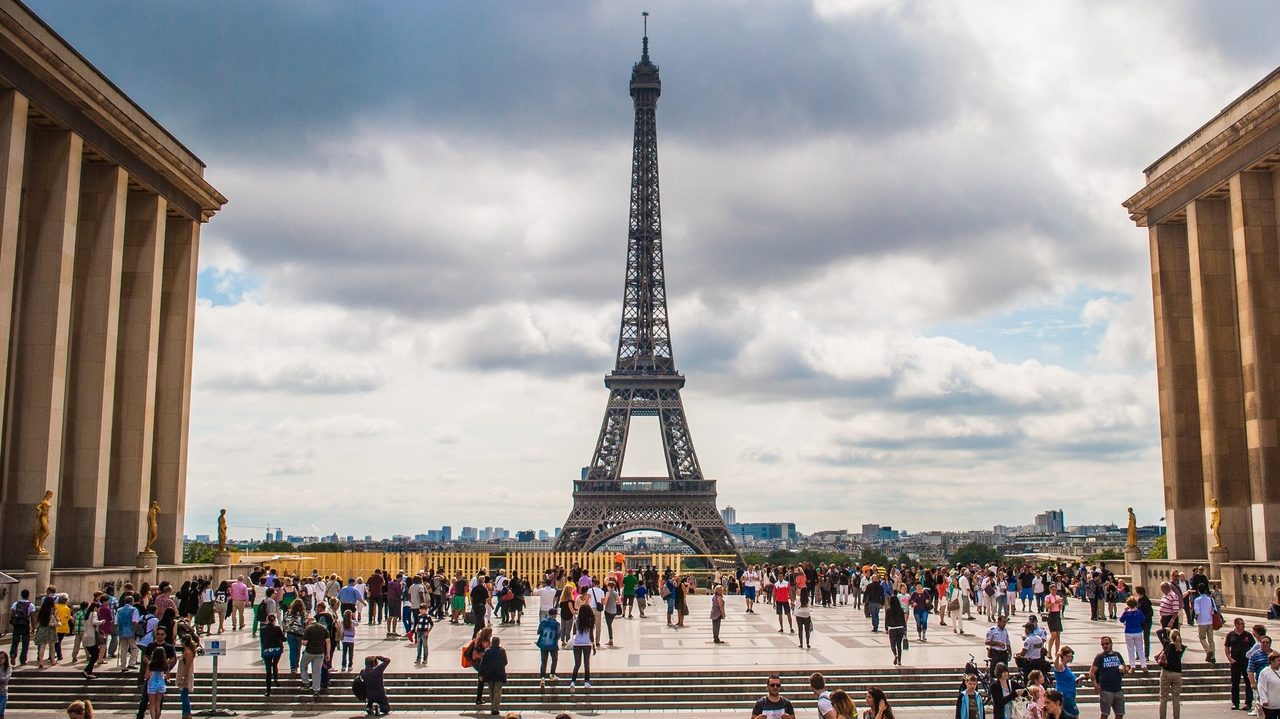
by Joanne Yu & Roman Egger
Full paper published by: Joanne Yu and Roman Egger, Information and Communication Technologies in Tourism 2021, https://link.springer.com/chapter/10.1007/978-3-030-65785-7_21
As a result of travel activities, overtourism has become a global issue. Even after the COVID-19 pandemic, the topic of overtourism would benefit localized overcrowding as a new occurrence in the tourism industry. To investigate tourists’ feelings when visiting overcrowded attractions, the analysis of online reviews has been recognized as a reliable source given the rich data it provides. In the digital area, reviews posted by tourists become critical in influencing one’s decision-making process. One typical example is TripAdvisor which enables tourists to consult reviews on any hotel, restaurant or attractions shared by other users.
Due to the unstructured nature of online data, topic modeling and sentiment analysis has gained their popularity. Topic modeling identifies the main topics of the reviews and is particularly suitable for exploratory studies. Sentiment analysis quantifies subjective information by natural language processing and computational linguistics. By taking overtourism (using Paris) as the research context, this study aims to uncover the most common issues when tourists visit overcrowded attractions and to reveal their feelings through text analytic techniques.
Data Collection and Data Preprocessing
All available English posts of the top 10 cultural-related attractions in Paris in TripAdvisor were extracted, resulting in a total of 140,712 posts published by any user as of the end of 2019. The attractions include Notre-Dame de Paris, Basilica of the Sacred Heart of Paris, Louvre Museum, Tour Eiffel, Centre Pompidou, Musée d’Orsay, City of Science and Industry, Museum of Natural History, Arc de Triomphe, and Sainte-Chapelle.
An open-source visual programming software, Orange 3, was applied for the following procedures. First, online posts were pre-processed. A list of stopwords was prepared to eliminate non-informative text. The remaining corpus was transferred to lowercase, where diacritics were transformed to the basic format. Next, text data was tokenized. All words were converted into their basic form, using lemmatization (e.g., traveling to travel).
Latent Dirichlet Allocation (LDA) Topic Model
This study applied LDA topic models to identify the underlying topics in an unstructured corpus such as customer reviews. Specifically, LDA views a document of text as a mixture of topics that disclose words with certain probabilities. However, due to the restriction of Orange 3 on the number of data instances, 5,000 posts were randomly selected for each attraction in Paris using a random selection in excel. LDA topic modeling was conducted to generate term clusters from the extracted reviews, which yielded 10 topics for each attraction based on the default setting of Orange 3. The degree of how a token contributes to a given review was revealed based on TF-IDF representation (term frequency-inverse document frequency).
Sentiment Analysis
In the next step, based on the identified topics, a lexicon-based sentiment analysis using the Vader algorithm was adopted to extract online users’ feelings based on the posts. Sentiments refer to feelings based on attitudes, emotions, and opinions; it determines whether an expression is positive, negative, or neutral. The results are presented by a numerical spectrum where −1 is the most negative, +1 is the most positive, and 0 suggests the neutral point.
Summary of the Results
LDA identifies 14 topics relevant to the issue of overcrowding and 10 general tourism-related topics. The table below presents the 14 overtourism topics and their corresponding average sentiment scores. The naming of the topic was based on the top keywords with the highest TF-IDF scores detected by LDA. For instance, the findings suggest that visitors felt most negatively about “safety and security”, “service and staff”, and “queues of customers”. Yet, the sentiment scores were higher regarding “social interaction”, “reputation”, and “overall atmosphere”. The detailed results can be found in our paper.
Topics | Keywords | Sentiment |
Safety and security | dangerous, suspicious, harass, scam, pickpocket, strangers, steal, group, busy, searched | 0.31 |
Queues of customers | wait, inform, queue, entrance, rough, long, fast, crowd, skip, stand | 0.51 |
Time to visit | hours, week, time, queue, days, nighttime, schedule, early, summer, weather | 0.67 |
Social interaction | many, groups, tourists, talk, gathering play, security, many, children, families | 0.73 |
Staff and service | staff, friendly, rude, helpful, English, nice, employees, confused, misunderstanding, refund | 0.34 |
Location and surroundings | places, landmark, Paris, city, location, distance, roads, community, cafes, area | 0.53 |
Service and facility | shop, restaurant, souvenir, expensive, staff, buy, pay, access, restroom, echo | 0.55 |
Time value of money | worthwhile, waiting, queue, crowds, wonderful, rewarding, special, affordable, reserve, value | 0.57 |
Visitor expectation | disappointing, crowded, small, few, overrated, wait, queue, bucket-list, lifetime, best | 0.59 |
Fee and ticket | price, free, ticket, enter, age, advance, early, group, cheap, pass | 0.62 |
Visitor recommendations | incredible, recommend, visit, return, must, advise, tip, overrated, impression, worth | 0.64 |
Emotional experience | disappointing, amazing, remarkable, unique, worth, awesome, breathtaking, boring, surprising, fun | 0.71 |
Reputation | famous, overrated, rewarding, unique, worth, overcrowded, icon, expect, nice, better | 0.80 |
Overall atmosphere | neighborhood, atmosphere, cozy, worth, views, outside, crowd, romantic, sparkles, light | 0.82 |
Unlike earlier research built upon existing measurements, this study takes one step further by exploratorily discovering the critical dimensions in managing tourist experiences at overcrowded attractions. Thus, this study contributes from a methodological angle by incorporating topic modelling technique and sentiment analysis to reveal tourists’ subjective perceptions. The technique applied in this study is beneficial to marketers who want to examine tourists’ feelings based on UGC elsewhere.
How to cite: Yu, J., & Egger, R. (2021). Tourist Experiences at Overcrowded Attractions: A Text Analytics Approach. In Information and Communication Technologies in Tourism 2021 (pp. 231-243). Springer, Cham.