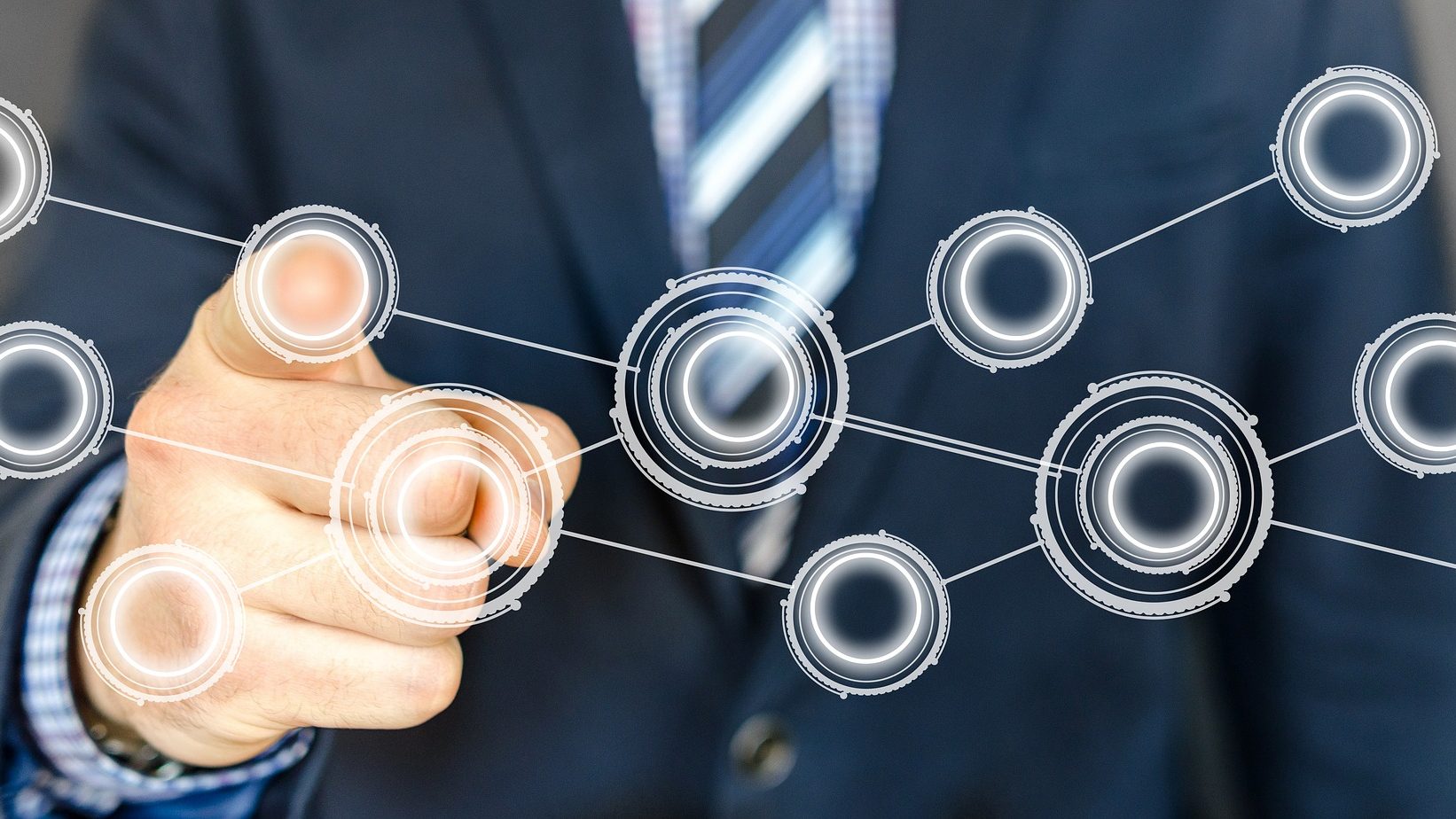
by ChengyuanZhangaShouyangWangbShaolongSuncYunjieWeib
Tourism Management Perspectives (2020), 35;
https://doi.org/10.1016/j.tmp.2020.100715
Utilizing a scientometric review of global trends and structure from 388 bibliographic records over two decades (1999-2018), this study seeks to advance the building of comprehensive knowledge maps that draw upon global travel demand studies. The study, using the techniques of co-citation analysis, collaboration network, and emerging trends analysis, identified major disciplines that provide knowledge and theories for tourism demand forecasting, many trending research topics, the most critical countries, institutions, publications, and articles, and the most influential researchers.
Especially, the increasing interest and output for big data and machine learning techniques in the field were visualized via comprehensive knowledge maps. On one hand, in big data era, a more reasonable integration of web-based data is the focus and direction of future research in forecasting tourism demand. While surveying contemporary topics and trends to understand tourists’ thinking and motives, several researchers have used search engine data and tourist emotion data as input variables to improve prediction accuracy.
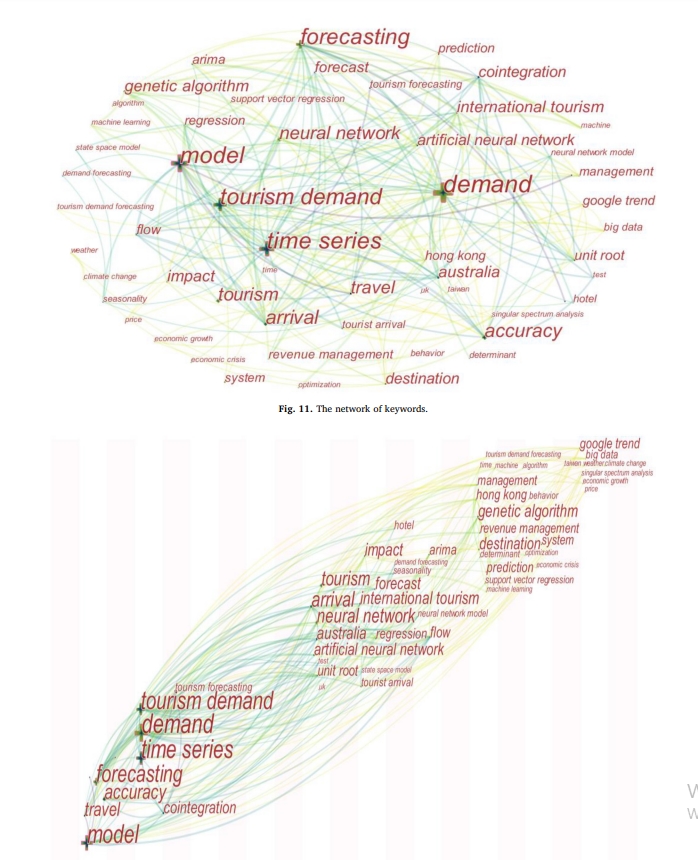
The studies revealed how web-based data from search engines, website traffic, and tourist emotion can be shaped into an exogenous variable to better forecast demand. Big data quality management has the potential to improve forecasting accuracy (Dergiades et al., 2018; Bokelmann & Lessmann, 2019), as well. Moreover, the influence of tourism-related events on demand, such as data applications generated by 5G technology, high-speed railway construction, policy measures, and thematic tourism (such as agritourism, parent-child travel) are, so far, untapped research directions. On the other hand, although artificial intelligence methods represented by neural network, genetic algorithms, and support vector regressions have been applied by scholars to tourism demand forecasting, deep learning has been less widely applied than in some other fields. Were deep learning’s application to tourism demand forecasting to reach full swing, there is a greater possibility for improving the prediction accuracy. Furthermore, based on the keyword clusters network, researchers have attempted to combine forecasts generated from different models to try to improve accuracy, but additional advanced individual forecasting methods and multiple forecasting horizons should be explored (Shen, Li & Song, 2011).
This research provides meaningful guidance for researchers, operators, and decision-makers who wish to improve the accuracy of tourism demand forecasting.